“像自动驾驶一样训练智能体完成蛋白进化”,华大智造研发团队Nature子刊发布强化学习算法 |
![]() |
[1] Yang, K. K., Wu, Z. Arnold, F. H. Machine-learning-guided directed evolution for protein engineering. Nat. Methods 16, 687-694 (2019). [2] Lin Z, Akin H, Rao R, et al. Evolutionary-scale prediction of atomic-level protein structure with a language model[J]. Science, 2023, 379(6637): 1123-1130. [3] Wang, J. et al. Scaffolding protein functional sites using deep learning. Science 377, 387-394 (2022). [4] Dauparas, J. et al. Robust deep learning based protein sequence design using ProteinMPNN. Science 378, 49-56 (2022). [5] Mnih, V. et al. Human-level control through deep reinforcement learning. Nature 518, 529-533 (2015). [6]Silver, D. et al. Mastering the game of go without human knowledge. Nature 550, 354-359 (2017). [7] Silver, D. et al. A general reinforcement learning algorithm that masters chess, shogi, and Go through self-play. Science 362, 1140-1144 (2018). [8] Feng, S., Sun, H., Yan, X.et al.Dense reinforcement learning for safety validation of autonomous vehicles.Nature 615, 620 627 (2023). [9] Degrave, J. et al. Magnetic control of tokamak plasmas through deep reinforcement learning. Nature 602, 414-419 (2022). [10] Schrittwieser J, Antonoglou I, Hubert T, et al. Mastering atari, go, chess and shogi by planning with a learned model[J]. Nature, 2020, 588(7839): 604-609. [11] Reed S, Zolna K, Parisotto E, et al. A generalist agent[J]. arXiv preprint arXiv:2205.06175, 2022 [12] Fawzi A, Balog M, Huang A, et al. Discovering faster matrix multiplication algorithms with reinforcement learning[J]. Nature, 2022, 610(7930): 47-53. [13] Perolat J, De Vylder B, Hennes D, et al. Mastering the game of Stratego with model-free multiagent reinforcement learning[J]. Science, 2022, 378(6623): 990-996. [14] Mankowitz D J, Michi A, Zhernov A, et al. Faster sorting algorithms discovered using deep reinforcement learning[J]. Nature, 2023, 618(7964): 257-263. [15] Schulman J, Wolski F, Dhariwal P, et al. Proximal policy optimization algorithms[J]. arXiv preprint arXiv:1707.06347, 2017. [16] Rives, A. et al. Biological structure and function emerge from scaling unsupervised learning to 250 million protein sequences. Proc. Natl. Acad. Sci. 118, e2016239118 (2021). [17] Meier, J. et al. Language models enable zero-shot prediction of the effects of mutations on protein function. Advances in Neural Information Processing Systems 34, 29287-29303 (NeurIPS, 2021). [18] Hie, B. L. et al. Efficient evolution of human antibodies from general protein language models and sequence information alone. Nat. Biotechnol. (2023). [19] Rao, R. et al. Evaluating protein transfer learning with TAPE. Advances in neural information processing systems 32 (NeurIPS, 2019). [20] Sinai, S. et al. AdaLead: A simple and robust adaptive greedy search algorithm for sequence design. Preprint at https://arxiv.org/abs/2010.02141 (2020). [21] Gonz lez J, Dai Z, Hennig P, et al. Batch Bayesian optimization via local penalization. In Proc. 38th International Conference on Machine Learning 648-657 (PMLR, 2016). [22] Angermueller, C. et al. Model-based reinforcement learning for biological sequence design. International conference on learning representations (ICLR, 2020). [23] Haarnoja T, Zhou A, Abbeel P, et al. Soft actor-critic: Off-policy maximum entropy deep reinforcement learning with a stochastic actor[C]//International conference on machine learning. PMLR, 2018: 1861-1870. [24] Brookes, D., Park, H. Listgarten, J. Conditioning by adaptive sampling for robust design. In Proc. 36th International Conference on Machine Learning 773-782 (PMLR, 2019). [25] Bryant, P. Elofsson, A. EvoBind: in silico directed evolution of peptide binders with AlphaFold. bioRxiv (2022). [26] Anishchenko, I. et al. De novo protein design by deep network hallucination. Nature 600, 547-552 (2021). [27] Yang, M., Pan, L. L., Liu, W. J., Wang, Y., et al. 新型桡足类荧光素酶突变体及其应用. PCT/CN2023/087445 (2023). [28] Zhang, W., Dong, Y. L., Li, J., Zheng, Y. Zhang, W. W. A Novel Gaussian Luciferase and Application. PCT/CN2021/144051 (2021). [29] Yeh A H W, Norn C, Kipnis Y, et al. De novo design of luciferases using deep learning[J]. Nature, 2023, 614(7949): 774-780.
医药网新闻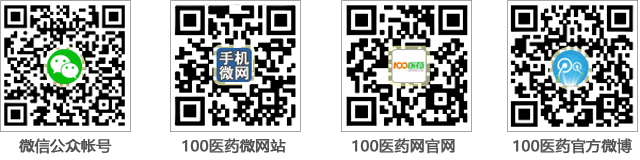
- 相关报道
-
- 89.5%改善率!NCR201细胞疗法实现帕金森病“关期”消失 (2025-06-08)
- 2025 ASCO:CheckMate 816/77T携两大LBA,试图解答能不能做“精准辅助治疗” ! (2025-06-07)
- Immunity:细菌抗肿瘤免疫疗法有益和有害作用的细胞机制 (2025-06-07)
- 程颖教授解读ES (2025-06-07)
- Nature:大脑不再是“免疫孤岛”!肠道、脂肪竟是T细胞的“大脑信使” (2025-06-07)
- 智康医疗突破性研究:基于血流动力学的颅内动脉瘤破裂风险评估 (2025-06-06)
- Nature Cancer:中科大刘连新团队等揭示相分离促进肝癌发展的新机制 (2025-06-06)
- 天津市三级病院九成以上已开明医保挪动付出,缴费不必再列队 (2025-06-06)
- BMJ Nutri Prev Health:警惕节食陷阱!低热量饮食或会加重机体的抑郁情绪 (2025-06-06)
- Nature Medicine:膀胱癌治疗里程碑!Sasanlimab联手BCG,无事件生存期显著延长,告别高复发时代! (2025-06-05)
- 视频新闻
-
- 图片新闻
-
医药网免责声明:
- 本公司对医药网上刊登之所有信息不声明或保证其内容之正确性或可靠性;您于此接受并承认信赖任何信息所生之风险应自行承担。本公司,有权但无此义务,改善或更正所刊登信息任何部分之错误或疏失。
- 凡本网注明"来源:XXX(非医药网)"的作品,均转载自其它媒体,转载目的在于传递更多信息,并不代表本网赞同其观点和对其真实性负责。本网转载其他媒体之稿件,意在为公众提供免费服务。如稿件版权单位或个人不想在本网发布,可与本网联系,本网视情况可立即将其撤除。联系QQ:896150040